Plan to Gather High-Quality Data from the Start
The success of any AI project hinges on the quality of the data collected from the outset. High-quality data forms the foundation for machine learning algorithms, guiding them to make informed decisions. If data is flawed – whether due to inaccuracies, incompleteness, or poor labeling – the resulting AI model will underperform. For example, a plant identification app trained solely on North American flora would fail to recognize plants from elsewhere, illustrating the critical "data cascade" effect.
To avoid such pitfalls, plan your data collection strategy carefully. This means thinking ahead about data types, ensuring the dataset is representative, and anticipating future use cases for enhanced performance across various contexts.
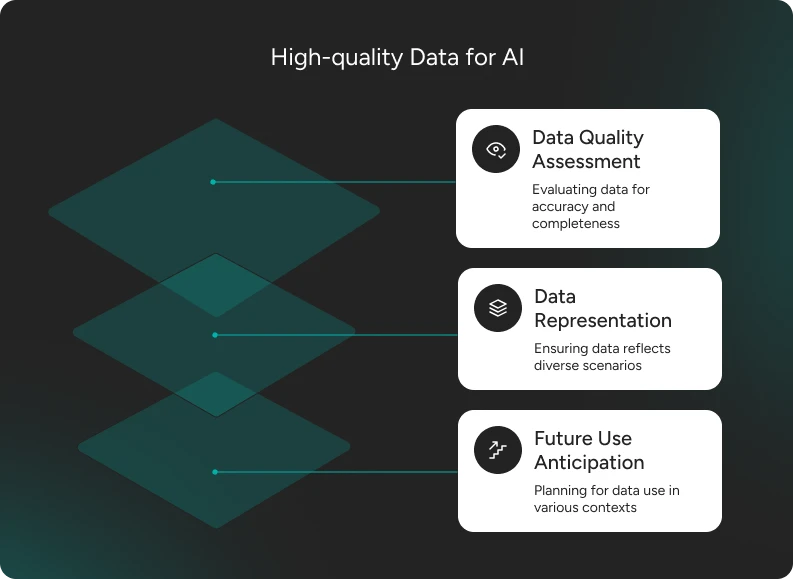
Translate User Needs into Data Needs
Translating user needs into data requirements is essential for developing effective AI solutions. It's crucial to understand user goals and define the specific data required to meet them.
Creating a solid data specification, similar to a product specification, ensures relevant data collection. For example, an app like "Plant Pal" requires detailed images, plant properties, and safety labels. Identifying and evaluating credible data sources, whether through partnerships or existing datasets, is vital to meet your project's needs. Additionally, consider threats like overfitting, where models become too tailored to training data, and underfitting, where models fail to capture key data relationships.
Source Your Data Responsibly
Sometimes it's tempting to pull data from the internet or user-generated content for its richness and diversity. However, this convenience comes with the responsibility to respect licenses, copyrights, and privacy laws.
Ethical sourcing not only safeguards against legal issues but also has a significant impact on the viability of AI initiatives. Vet datasets thoroughly for biases and errors, aiming to mix clean baseline data with a touch of real-world complexity. Remember to protect personal data and comply with privacy laws like GDPR, potentially anonymizing sensitive information through aggregation or redaction techniques.
Prepare and Document Your Data
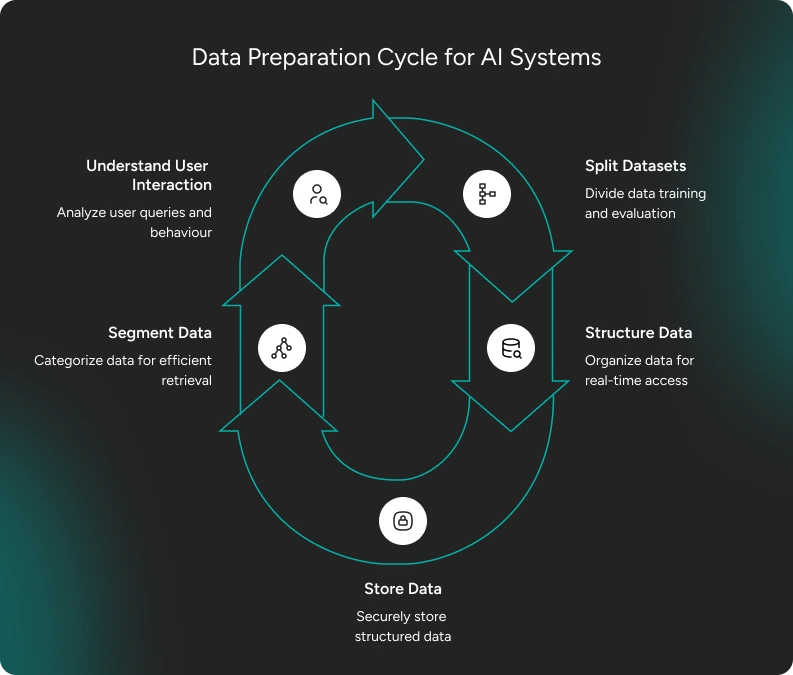
Data preparation is crucial in both traditional AI training and in developing products powered by Retrieval Augmented Generation (RAG). While the traditional approach focuses on refining data for model training, RAG requires structuring data for real-time access. Ensure datasets are split effectively for learning and evaluation.
As we shift to RAG, focus on storing, structuring, and segmenting data to empower AI systems to retrieve information accurately. Understanding user interaction with data and anticipated queries is crucial for creating intelligent systems that navigate resources seamlessly.
Design for Labelers & Labeling
Accurate data labeling is fundamental for meaningful machine learning outputs. Provide labelers with clear instructions and tools to ensure high-quality, unbiased data. Diversity among labelers is key to reducing bias, improving data quality, and accommodating cultural nuances.
Effective labeling tools feature intuitive interfaces, clear instructions, and error detection. Incorporate inclusivity and accessibility, ensuring the process accommodates various environments and devices. Regular reliability checks through statistical measures can highlight issues and optimize design.
Tune Your Model
Once the data is gathered, prepared, and has a functioning model, the next step is optimization. The question then becomes: How can we enhance it even further? This is where model tuning comes into play.
It involves adjusting available parameters to refine the model's output, ensuring it aligns with predefined user needs and success metrics. For a comprehensive evaluation, it’s valid to incorporate user feedback alongside tools like the What-If Tool. Interactions may be monitored through dashboards to track and improve user experience. It's also important to note that tuning goes beyond technical adjustments, as it focuses on delivering an optimal user experience.
UX Designers are essential in aligning model accuracy with user satisfaction, interpreting behavior to refine models, and defining success metrics. Fine-tuning adjusts models to improve performance in specific contexts, with UX Designers ensuring alignment with user expectations and real-world demands.
Guiding AI Development with Ethics and Quality
Navigating the complexities of AI development requires meticulous attention to data quality, ethical sourcing, thorough preparation, strategic labeling, and continuous model tuning. By aligning your AI systems with user needs and ethical standards, and by integrating thoughtful design and feedback, you can create AI solutions that not only perform technically but also resonate with real-world users. As AI continues to evolve, staying attuned to these best practices will ensure your AI projects drive meaningful innovation and results.