The STX Data difference
In today's data-driven world, companies in various industries face significant data challenges that can hinder growth. At STX Next, we understand these hurdles and offer tailored data engineering solutions to help you succeed. We provide:
Unified Data Platform
AI-Powered Analytics
Real-Time Event Handling
Customer
testimonial
The most important and best thing that I've experienced is communication styles. The communication has been flawless. Przemysław (Product Owner) has been amazing to work with, answering my questions. I've been able to work with several of the developers as well whenever I have challenges that I come across. And everybody has been willing to help and to try to help me solve the issue that I'm experiencing.
Why
STX Next
?
Fact no.1
Customizable
Data
Solutions
Fact no.2
Efficient
Delivery
Capabilities

Fact no.3
Collaborative
Approach
Fact no.4
Comprehensive
Support
The
importance
of
Data Engineering
Data Engineering is essential for making data accessible and usable for data scientists and Business Intelligence developers. It involves building and maintaining data lakes, data warehouses, and data pipelines to analyze raw data and create predictive models, guaranteeing cost-efficiency, and making data-driven, informed choices.

Top
challenges
in Data Engineering
While data engineering offers substantial benefits, businesses often encounter several key challenges. Here’s how STX Next can help you overcome these obstacles:
challenge no.1
Understanding Big Data
Managing and comprehending large volumes of data can be challenging, making it hard to capture the details you need.
Our data engineering services include robust data pipelines and advanced data analytics to simplify big data management and enhance clarity.
challenge no.2
Data Growth Issues
We focus on cost-efficiency, ensuring you get the performance you need at a reasonable price.
Our partnerships with major Cloud providers also help you secure discounts or dedicated funding for your projects.
challenge no.3
Data Integration
Integrating data from multiple sources is crucial for comprehensive analytics.
Our seamless data integration solutions combine data from various heterogeneous sources, coming in different shapes or forms, into a unified platform.
challenge no.4
Data Security
Protecting data from breaches and ensuring compliance is vital.
With advanced data governance and master data management techniques, we enhance data security and ensure your business meets regulatory standards.
challenge no.5
Real-Time Data Processing
Capturing and processing data in real-time is crucial for timely decision-making.
We employ high-speed data streaming and real-time analysis tools to ensure you have the most current data for making informed decisions promptly.
Technologies
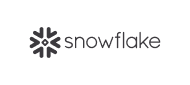



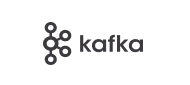
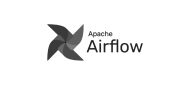
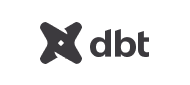
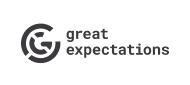
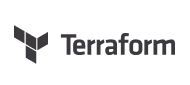
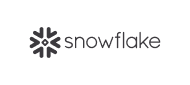



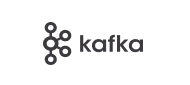
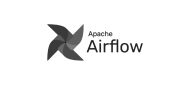
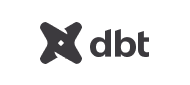
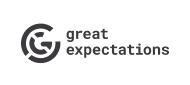
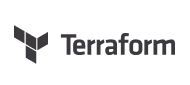
Data Engineering
FAQs
How does Data Engineering work in different industries?
Data Engineering is like the behind-the-scenes work that makes data useful for businesses across different industries. Let's break it down with some examples:
Healthcare
In healthcare, data engineers gather and process patient records, research data, and operational data into a data lake. Through data processing and data analytics, hospitals can improve patient care, manage resources better, and comply with regulatory standards with effective data governance.
Finance Industry
Data engineering services help banks and financial institutions manage vast amounts of data. They create data pipelines to move and transform data from various sources into a centralized data warehouse. This data integration and management ensure that the information is accurate and readily available for data analytics and data science which helps in risk assessment, fraud detection, and customer insights. Additionally, real-time data streaming and analytical systems improve traders' efficiency and decision-making in their daily operations.
Manufacturing
Manufacturers use data engineering to integrate data from production lines, quality control, and supply chains into a data warehouse. This facilitates data analytics for predictive maintenance, which prevents machine failures and downtime. Data engineers also ensure data quality and efficiency in data processing.
Marketing and Advertising
These industries rely heavily on data engineering to gather information from multiple data sources like social media, email campaigns, and website analytics. Suitable infrastructure supports data analytics and machine learning models in delivering targeted ads and personalized marketing strategies.
What is Data Mesh?
Data Mesh is a modern approach in data engineering that decentralizes data management, moving away from traditional data lakes and data warehouses. Instead of having a central team handle all data, it distributes responsibility across different business domains like marketing, sales, and finance. It consists of:
- Domain Ownership: Each domain manages its data sources and ensures high data quality and effective data governance. A data engineer in each domain handles data integration and data management.
- Data as a Product: Domains treat their data as products, ensuring it's well-maintained and documented. This makes it easier for others to use and derive meaningful insights.
- Self-Serve Data Platform: A shared platform provides tools for data processing, and other infrastructure needs, reducing reliance on a centralized IT department.
- Federated Governance: There’s a balanced governance model to enforce organization-wide policies, ensuring data security and compliance while allowing domain autonomy.
This decentralized approach helps businesses scale their data architecture, enhance agility, and quickly gain valuable insights from their data analytics and data science efforts.
What’s the difference between Data Engineering and Data Science?
Data Engineering is about building and managing data systems, while Data Science focuses on analyzing and interpreting data to drive business decisions. The key characteristics include:
Data Engineering:
- Data Engineers build and maintain data infrastructure like data lakes and data warehouses.
- Focuses on data integration, data quality, and data processing.
- Responsible for data ingestion, data transformation, and data governance.
Data Science:
- Data Scientists analyze data to extract valuable insights.
- Utilizes data analytics, machine learning, and advanced analytics to find patterns and make predictions.
- Relies on infrastructure built by data engineers and presents findings via data visualization.
What is a Data Pipeline?
A Data Pipeline is a series of steps that transform raw data into useful insights for your business. Such methodology ensures efficient data processing and data management, supports high-quality data governance, and provides a solid foundation for data analytics and data science. A Data Pipeline requires the following key components:
- Data Ingestion: Collects data from various data sources.
- Data Transformation: Cleans and converts data, ensuring high data quality.
- Data Storage: Stores data in data warehouses or data lakes.
- Data Integration: Combines data from different sources.
- Data Analysis: Prepares data for data analytics and data science to extract actionable insights.
How do you secure data and privacy with Data Engineering?
Data Engineering combines technologies and policies to secure data and protect privacy, ensuring that sensitive information remains safe and compliant. This protects data quality and integrity, safeguards against data breaches, and maintains customer trust and privacy.
Securing Data and Privacy in Data Engineering:
- Data Governance: Establish rules for data access, management, and protection.
- Data Encryption: Protect data both at rest and in transit through encryption.
- Access Control: Use role-based access control (RBAC) to restrict data access to authorized users.
- Data Masking: Hide or obfuscate sensitive information to protect privacy.
- Logging and Monitoring: Continuously monitor data access and usage to detect suspicious activities.
- Compliance: Ensure practices meet regulations like GDPR, HIPAA, and CCPA.
- Data Anonymization: Anonymize data for data analytics and data science to protect identities.
- Regular Audits: Conduct security audits to identify and fix vulnerabilities.
Our customers love to work with us