Two of our Expert Python Developers, Radosław Jankiewicz and Tomasz Maćkowiak, have prepared everything you need to get started. If you’re looking for a practical introduction to Machine Learning in Python, look no further. ML is a subset of Artificial Intelligence, and Python is also widely used in deep learning projects.
What You'll Learn in this Machine Learning Tutorial in Python
Machine Learning is definitely on the rise nowadays. The ability of computers to learn from examples instead of operating strictly according to previously written rules is an exciting way of solving problems.
Python is the most popular language for Machine Learning and data science. In this article we will show the basic tool chain for implementing ML in Python.
We will explain:
- how to load a data set
- the role of sample data in training and testing ML models
- how to run a ML algorithm on the data
- how to assess the performance of the algorithm
- the importance of understanding different ML models
…all in just few lines of Python code!
But first, a disclaimer. We want to show you in practice how to take your first steps with ML without getting drowned in theory. So we will only give you the ‘need-to-know’ of what Machine Learning is.
We will not explain how the algorithm works. We will not show how to choose the right algorithm for your problem. Nor will we present how to optimize the parameters of the algorithm.
We will concentrate on the basics and we are going to go over the process of Machine Learning on a concrete example from A (getting data) to Z (evaluating the performance [accuracy] of the created model).
We assume that the reader has a rough knowledge about what Machine Learning is about and that he knows Python already. Having basic knowledge of Python programming will facilitate easier comprehension of the tutorial content.
We hope that by the end of this article you will be able to see why Python is the number one choice for this domain. Python libraries play a crucial role in implementing ML models, providing foundational tools to explore various data science challenges. These libraries help in building and applying different models, such as decision trees and regression methods, to solve real-world data problems.
Definition and Importance of ML
Machine learning is a subset of artificial intelligence that focuses on developing algorithms and statistical models that enable computers to learn from data and make predictions or decisions without being explicitly programmed. This means that instead of following a set of pre-defined rules, Machine Learning systems improve their performance by learning from past data. The importance of ML lies in its ability to automate tasks, extract valuable insights from vast amounts of data, and enhance decision-making processes. In today’s world, where data is generated at an unprecedented rate, Machine Learning has become a crucial tool for organizations to gain a competitive edge and drive innovation.
How Machine Learning Works
ML works by using algorithms to analyze and learn from data. The process begins with training a model on a dataset, which involves feeding the model input data along with the corresponding output labels. This training enables the model to identify patterns and relationships within the data. Once trained, the model can make predictions or decisions on new, unseen data. The accuracy of these predictions depends on the quality and quantity of the training data, as well as the choice of algorithm. Machine learning can be applied to various tasks, including classification, regression, clustering, and dimensionality reduction, making it a versatile tool for solving a wide range of problems.
Our Problem
The goal of this machine learning tutorial is to show ML on an approachable example. An important issue you need to solve at the beginning is acquiring a dataset.
Luckily, there are large datasets publicly available for use and they are extremely useful for starting your adventure in Machine Learning.
For this article we chose a problem that can be researched using a public dataset (more information about acquiring it later). Python machine learning is crucial for implementing the techniques needed to solve this problem.
The example problem we would like to tackle with Machine Learning is the following:
Based on a person’s attributes (like age, working hours, industry sector, etc.), predict whether the person has a high salary or not (whether they earn more or less than 50,000 USD per year).
This problem is a classification problem. We want to categorize the population into two classes: high-income and low-income. Since there are only two classes and each person belongs to exactly one class we call it a binary classification problem.
Customer segmentation is another example of a classification problem that can be solved using Machine Learning.
In other words, for each person we are trying to determine whether they belong to the low-income class or not.
What is the ML Process? A High-Level ML Overview
The process of Machine Learning can be split into the following steps:
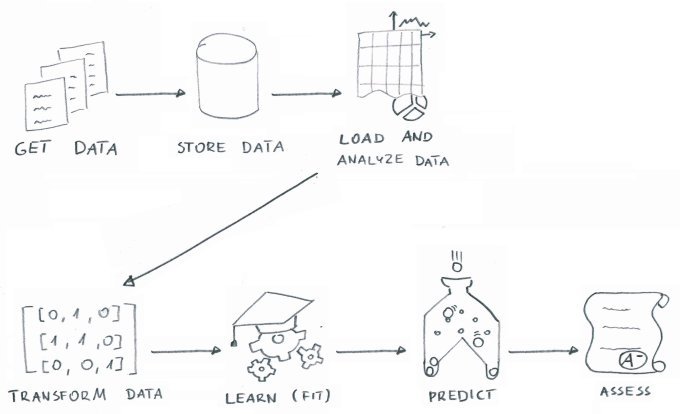
Get Data
Acquire a big enough dataset (including labels or answers to your problem).
Store Data
Store the acquired data in a single location for easy retrieval.
Load and Perform Data Analysis
Load your dataset from storage and do basic data analysis and visualization.
Transform Data
Machine Learning requires purely numeric input, so you need to transform the input data.
Learn (Fit)
Run the labeled data through a Machine Learning algorithm yielding a model.
Predict
Use the model to predict labels for data that the model did not see previously.
Assess
Verify the accuracy of predictions made by the model.
Introduction to Machine Learning Models
ML models are mathematical representations of real-world systems that can be used to make predictions or decisions. There are several types of ML models, each suited to different tasks. Supervised learning models are trained on labeled data, where the input data is paired with the correct output. These models are used for tasks such as classification, where the goal is to categorize data into predefined classes, and regression, where the goal is to predict continuous values. Unsupervised learning models, on the other hand, are trained on unlabeled data and are used for tasks such as clustering, where the goal is to group similar data points, and dimensionality reduction, where the goal is to reduce the number of features in the data. Reinforcement learning models are trained using feedback from the environment and are used for tasks such as game playing and robotics, where the model learns to make a sequence of decisions to achieve a goal.
Types of Machine Learning
Machine Learning can be broadly classified into several types, each with its unique approach to learning from data:
- Supervised Learning: In supervised learning, the algorithm is trained on labeled data, where the correct output is already known. The goal is to learn a mapping between input data and the corresponding output labels. This type of ML is commonly used for tasks such as classification and regression.
- Unsupervised Learning: Unlike supervised learning, unsupervised learning deals with unlabeled data. The algorithm’s goal is to discover patterns or relationships within the input data. Clustering and dimensionality reduction are typical applications of unsupervised learning.
- Semi-Supervised Learning: This type of ML combines both labeled and unlabeled data for training. It leverages the small amount of labeled data to guide the learning process, making it useful when acquiring labeled data is expensive or time-consuming.
- Reinforcement Learning: In reinforcement learning, the algorithm learns by interacting with an environment and receiving feedback in the form of rewards or penalties. This type of learning is often used in robotics, game playing, and other scenarios where decision-making is crucial.
- Deep Learning: A subfield of ML, deep learning involves the use of neural networks with multiple layers to learn complex patterns in data. Deep learning has been particularly successful in areas such as image recognition, natural language processing, and speech recognition.
Understanding these types of Machine Learning helps in selecting the appropriate approach for different data-driven problems.
Common Machine Learning Algorithms
There are several common machine learning algorithms, each with its own strengths and applications. Linear regression is a supervised learning algorithm used for regression tasks, where the goal is to predict a continuous value based on input features. Logistic regression, another supervised learning algorithm, is used for classification tasks, where the goal is to categorize data into predefined classes. Decision trees and random forests are versatile supervised learning algorithms that can be used for both classification and regression tasks. Decision trees work by splitting the data into branches based on feature values, while random forests combine multiple decision trees to improve accuracy and reduce overfitting. Support vector machines are powerful supervised learning algorithms used for classification and regression tasks, which work by finding the optimal hyperplane that separates data points of different classes.
Getting Data
In order to start the Machine Learning process you need to possess a set of data to be used for training the algorithm.
It's very important to ensure that the source of data is credible, otherwise you would receive incorrect results, even if the algorithm itself is working correctly (following the garbage in, garbage out principle).
Understanding different data types and main categories is crucial for proper data analysis. Data can be categorized into three main types: numerical, categorical, and ordinal. Numerical data includes values that represent measurable quantities, categorical data represents distinct groups or categories, and ordinal data represents categories with a meaningful order. Recognizing these types helps in selecting the appropriate techniques for data analysis.
The second important thing is the size of the dataset. There is no straightforward answer for how large it should be. The answer may depend on many factors, for example:
- the type of problem you're looking to solve,
- the number of features in the data,
- the type of algorithm used.
Fortunately, it shouldn't be difficult to find a ready-made dataset for your example project.
For starters you may use one of built-in datasets provided by scikit-learn package.
A popular choice is the Iris flower dataset that consists of data on petal and sepal length for 3 different types of irises (Setosa, Versicolour, and Virginica), stored in a 150×4 numpy.ndarray:
from sklearn import datasets >>> iris = datasets.load_iris() >>> print(iris.DESCR) Iris Plants Database ====================
Notes —– Data Set Characteristics: :Number of Instances: 150 (50 in each of three classes) :Number of Attributes: 4 numeric, predictive attributes and the class :Attribute Information: - sepal length in cm - sepal width in cm - petal length in cm - petal width in cm - class: - Iris-Setosa - Iris-Versicolour - Iris-Virginica …
iris.data[:5] array([[ 5.1, 3.5, 1.4, 0.2], [ 4.9, 3. , 1.4, 0.2], [ 4.7, 3.2, 1.3, 0.2], [ 4.6, 3.1, 1.5, 0.2], [ 5. , 3.6, 1.4, 0.2]])
Another good source of interesting publicly available datasets is the UC Irvine Machine Learning Repository which contains a vast collection of datasets used throughout the ML community.
For the purposes of this article we chose the Adult Data Set that contains 48,842 records extracted from the US 1994 Census database. Each record contains 14 attributes:
- age – integer,
- workclass – categorical values (‘Private', ‘Self-emp-not-inc', ‘Self-emp-inc', ‘Federal-gov', …),
- fnlwgt – integer,
- education – categorical (‘Bachelors', ‘Some-college', ‘11th', ‘HS-grad', …),
- education-num – integer,
- marital-status – categorical (‘Married-civ-spouse', ‘Divorced', ‘Never-married', ‘Separated', …),
- occupation – categorical (‘Tech-support', ‘Craft-repair', ‘Other-service', ‘Sales', …),
- relationship – categorical (‘Wife', ‘Own-child', ‘Husband', ‘Not-in-family', …),
- race – categorical (‘White', ‘Asian-Pac-Islander', ‘Amer-Indian-Eskimo', ‘Other', …),
- sex – categorical (‘Female', ‘Male'),
- capital-gain – integer,
- capital-loss – integer,
- hours-per-week – integer,
- native-country – categorical (‘United-States', ‘Cambodia', ‘England', ‘Puerto-Rico', …).
For each record we also get the classification label (< =50k or >50k – information about the yearly salary bracket).
Based on this dataset we're going to train a classification algorithm to be able to predict whether a person with a given set of attributes earns more or less than 50 thousand dollars per year.
Training Data and Test Data
After training your model, you will surely want to know if it is good enough at solving the problem in the real world.
To correctly measure the accuracy of your model, you need to validate it against a new set of data – different than the set your were training it with.
Thus, before using the collected dataset for training your algorithm, you should split it into a subset which will be used for the training process (training set) and a subset that will be used for validating the accuracy of the algorithm (test set).
In practice, you should devote 20-30% of your collected dataset for validation purposes (test set).
Suppose you have a matrix of input data X and a vector of corresponding expected results y. You can use a simple utility function: sklearn.model_selection.train_test_split to split it into a train and test subsets with the given proportion:
from sklearn.model_selection import train_test_split
X_train, X_test, y_train, y_test = train_test_split(X, y, test_size=0.25)
For our example problem we don't have to split the dataset on our own. The Adult Data Set collection we chose already consists of two separate files:
- training set – adult.data (32,561 records)
- test set – adult.test (16,281 records)
Loading Data with Pandas
Disclaimer: we omit the description of loading data from text files downloaded from the UC Irvine Machine Learning Repository into a SQLite database because that is outside the scope of this article. You can still read our solution yourself in the Complete listing section.
Once you have your data stored in a single location you should load them into a tool that will allow you to analyze it easily, slice'n'dice them and later on use them with your Machine Learning algorithms.
The Python pandas package is a great tool for that.
Out of the box it allows you to read your data from a variety of formats:
- flat files such as CSV, JSON, HTML,
- binary formats including Excel and pickle,
- relational databases,
- cloud (Google Big Query),
- and others.
Below we present an example of reading data from an SQL database through SQLAlchemy.
import os.path import pandas from sqlalchemy import create_engine
def read_data_frame(): DB_FILE_PATH = os.path.join(os.path.dirname(__file__), 'data.sqlite') TABLE_NAME = 'adult' engine = create_engine(f'sqlite:///{DB_FILE_PATH}') with engine.connect() as conn: with conn.begin(): return pandas.read_sql_table(TABLE_NAME, conn, index_col='id')
The data is read as a pandas DataFrame object. The object contains information about properties (columns) in the data:
>>> data_frame.columns Index(['age', 'workclass', 'fnlwgt', 'education', 'education_num', 'marital_status', 'occupation', 'relationship', 'race', 'sex', 'capital_gain', 'capital_loss', 'hours_per_week', 'native_country', 'classification'], dtype='object')
You can view a data record:
>>> data_frame.iloc[0] age 39 workclass State-gov fnlwgt 77516 education Bachelors education_num 13 marital_status Never-married occupation Adm-clerical relationship Not-in-family race White sex Male capital_gain 2174 capital_loss 0 hours_per_week 40 native_country United-States classification <=50K Name: 1, dtype: object
You can view the data column by column:
>>> data_frame.workclass id 1 State-gov 2 Self-emp-not-inc 3 Private 4 Private 5 Private 6 Private 7 Private 8 Self-emp-not-inc 9 Private 10 Private ... 32552 Private 32553 Private 32554 Private 32555 Private 32556 Private 32557 Private 32558 Private 32559 Private 32560 Private 32561 Self-emp-inc Name: workclass, Length: 32561, dtype: object
You can quickly get a summary of value counts for a specific column:
>>> data_frame.workclass.value_counts() Private 22696 Self-emp-not-inc 2541 Local-gov 2093 ? 1836 State-gov 1298 Self-emp-inc 1116 Federal-gov 960 Without-pay 14 Never-worked 7 Name: workclass, dtype: int64
The pandas library enables you to group, filter, transform your data and much, much more.
Data Visualization with Matplotlib
Before you start modeling the data it may be very beneficial to visualize it. It will let you better understand the nature of the data you're going to work with. You might find relationships and patterns between input values which will help you get better results.
Data visualization may also help you to pre-validate the input data. For example, you would expect that most people work 40 hours a week. In order to examine if your assumption is correct you could draw a histogram chart. You can do it quickly using the matplotlib plotting library integrated with your pandas DataFrame:
import matplotlib.pyplot as plt
data_frame.hours_per_week.plot.hist(bins=30) plt.show()
It should display the following chart:

Data visualization is also beneficial in natural language processing tasks to understand text data better.
Hours per Week Histogram
A quick look at the generated chart confirms that your assumption was correct.
Suppose you would like to see how age and the number of hours worked per week correlate with earnings. For that your can make matplotlib draw a scatter plot of your data:
import numpy as np
colors = np.where(data_frame.classification == '>50K', 'r', 'k') plot = data_frame.plot.scatter(x='age', y='hours_per_week', s=10, c=colors) plot.figure.show()
As a result you receive a chart showing correlation between values from two columns of your collection (age and number of hours worked per week) where the red dots represents people whose yearly earnings are higher and black dots lower than $50,000:
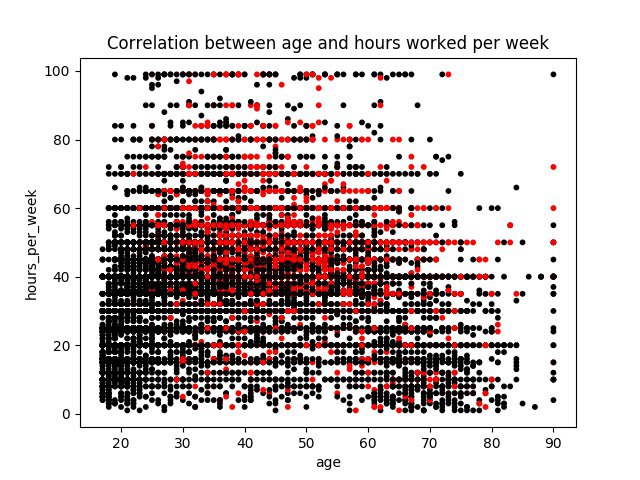
Scatter Plot Example
You can see that the density of red dots is higher in the area represented by samples of people between 30 and 60 years where the hours worked per week is above 40.
As you can see matplotlib is a powerful and easy to use library which can be very useful for visualizing the processed data. Moreover, it's nicely wrapped by Series and DataFrame objects which are used for representing datasets in pandas library, which makes plotting different kinds of charts even more handy.
Transforming Data with Sklearn-Pandas
Mapper
The Machine Learning algorithm expects only numerical values as input. To be exact, it expects a numpy low-level matrix of numerical data.
The data we loaded earlier is stored in a pandas DataFrame. To transform the DataFrame into the numpy array we need, we can use DataFrameMapper from sklearn-pandas – a library that bridges the gap between pandas and sklearn.
The mapper allows us to select which data attributes (columns) we want to use for Machine Learning and what transformations should be performed for each attribute. Each column can have one or multiple transformations applied in turn:
import sklearn.preprocessing from sklearn_pandas import DataFrameMapper
mapper = DataFrameMapper([ (['age'], sklearn.preprocessing.StandardScaler()), # single transformation ('sex', sklearn.preprocessing.LabelBinarizer()), # single transformation ('native_country', [ # multiple transformations sklearn.preprocessing.FunctionTransformer( native_country_generalize, validate=False ), sklearn.preprocessing.LabelBinarizer() ]), ... ])
If the column does not need any transformations use None in the configuration for that attribute. Attributes not mentioned in the mapper configuration will not be used in the mapper's output.
In our data we have some numerical attributes (for example age) as well as some string enumerations (for example sex, marital_status).
Scaling numerical values
It is a good practice to scale all numerical values to a standard range to avoid problems when one attribute (for example capital_gain) would outweigh another's importance (for example age) due to its values' higher order of magnitude. We can use sklearn.preprocessing.StandardScaler to scale the values for us.
Transforming enumerations
Enumerations are a more complex case. If the enumeration only has 2 possible values:
id sex 1 male 2 female 3 female 4 male
we can convert the column to a boolean flag column:
id sex 1 0 2 1 3 1 4 0
If the enumeration has more values, for example:
id marital_status 1 Married 2 Never-married 3 Divorced 4 Never-married 5 Married 6 Never-married 7 Divorced
then we can transform it into a series of boolean flag columns, one for each possible enumeration value:
id
marital_status_Married
marital_status_Never-married
marital_status_Divorced
1
1
0
0
2
0
1
0
3
0
0
1
4
0
1
0
5
1
0
0
6
0
1
0
7
0
0
1
sklearn.preprocessing.LabelBinarizer can handle both of the scenarios listed above.
Complex transformations
Sometimes we want to run a more advanced transformation on data including applying some business logic. In our data the attribute native_country has 42 possible values, though 90% of the records contain the value United-States.
To avoid creating 42 new columns, we would like to reduce the column to contain a smaller set of values: United-States and Other for the 10% remaining records. We can use sklearn.preprocessing.FunctionTransformer to achieve this:
import numpy import functools
def numpy_map(callback): @functools.wraps(callback) def numpy_map_wrapper(X): return numpy.array([callback(x) for x in X]) return numpy_map_wrapper
@numpy_map def native_country_generalize(x): return 'US' if x == 'United-States' else 'Other'
mapper = DataFrameMapper([ ... ('native_country', [ sklearn.preprocessing.FunctionTransformer( native_country_generalize, validate=False ), sklearn.preprocessing.LabelBinarizer() ]) ])
Notice how we are still running the output of the FunctionTransformer through LabelBinarizer to convert new enumerations to boolean flags.
Features
The DataFrameMapper converts our pandas DataFrame into a numpy matrix of features. A feature is a single input to our Machine Learning algorithm.
As you could see, one column of our original data can correspond to more than one feature (in the case of enumerations).
If you would like to preview the output that the mapper is producing, you can run it on the training data inputs:
>>> data = mapper.fit_transform(train_X) >>> data array([[ 0.03067056, 1. , 0. , ..., -0.21665953, -0.03542945, 1. ], [ 0.83710898, 0. , 0. , ..., -0.21665953, -2.22215312, 1. ], [-0.04264203, 0. , 0. , ..., -0.21665953, -0.03542945, 1. ], ..., [ 1.42360965, 0. , 0. , ..., -0.21665953, -0.03542945, 1. ], [-1.21564337, 0. , 0. , ..., -0.21665953, -1.65522476, 1. ], [ 0.98373415, 0. , 0. , ..., -0.21665953, -0.03542945, 1. ]]) >>> data.dtype dtype('float64')
You can see that the mapper produced a two-dimensional numpy matrix of floating point values. This is the format of input that the Machine Learning algorithm expects.
However, this data is just a collection of numbers. It does not store information about column names or enumeration values. In other words, the data in this format is hardly human readable. It would be difficult to analyze the data in this state. That's why we'd rather use pandas to load and play with the data, and execute this transformation only just before running the algorithm.
Setting Up a Machine Learning Environment in Python
To set up a Machine Learning environment in Python, you need to install several essential libraries. NumPy is a fundamental library that provides support for large, multi-dimensional arrays and matrices, along with a collection of mathematical functions to operate on these arrays. Pandas is another crucial library that offers data structures and data analysis tools, making it easy to manipulate and analyze data. Scikit-learn is a comprehensive library that provides a wide range of ML algorithms and tools for model training, evaluation, and deployment. You can install these libraries using pip, the package installer for Python, with the following commands: pip install numpy pandas scikit-learn
Once you have installed these libraries, you can start building and training ML models using Python. These libraries provide a robust foundation for implementing various Machine Learning methods, from data preprocessing and visualization to model training and evaluation, enabling you to tackle a wide range of data science challenges. Python machine learning tutorials and resources emphasize Python's significance and its libraries in the field, catering to both beginners and experienced practitioners looking to enhance their skills.
Understanding Python’s Role in Machine Learning
Python plays a vital role in machine learning due to its simplicity, flexibility, and extensive libraries. Python’s syntax is easy to learn, making it an ideal language for both beginners and experts. This simplicity allows developers to focus on building machine learning models rather than getting bogged down by complex syntax.
One of the key strengths of Python in machine learning is its vast collection of libraries. Libraries such as NumPy and pandas provide efficient data manipulation and analysis capabilities, which are essential for preprocessing data before feeding it into machine learning models. For instance, NumPy offers support for large, multi-dimensional arrays and matrices, while pandas provides data structures and tools for data analysis.
When it comes to building machine learning models, Python’s scikit-learn library is a powerhouse. It offers a wide range of algorithms, including decision trees, random forests, and support vector machines, making it easy to implement and experiment with different models. Scikit-learn also provides tools for model evaluation, helping developers select the best model for their data.
Python’s simplicity and flexibility extend to the deployment of machine learning models. Whether you’re integrating a model into a web application or deploying it on a mobile device, Python’s versatility makes the process straightforward. This ease of deployment ensures that machine learning solutions can be quickly and efficiently brought to production.
In summary, Python’s role in machine learning can be encapsulated in its ability to handle data preprocessing, model building, evaluation, and deployment with ease. Its extensive libraries and supportive community make it an ideal choice for machine learning projects.
Predicting using Machine Learning Models
The classification model produced as a result of the training process can be now used to predict the classification on the test set DataFrame or possibly totally new data out in the wild.
test_X = test_data_frame[test_data_frame.columns.drop('classification')] predictions = model.predict(X=test_X)
Evaluating the model
The last step you should take is model evaluation. This will tell you how accurate the predictions made by the trained model are.
As you might notice, the evaluation process is executed on the previously extracted test set (test_X, test_y) which was not seen by the model earlier, during the training process.
You should never evaluate the model on the train set, because the results obtained would not translate into real world applications (in that way you wouldn't be able to verify if your model is able to make generalizations).
There are a couple of metrics that let you evaluate the accuracy of your model. The most basic one is sklearn.metrics.accuracy_score which represents a ratio of all correctly predicted values to all processed samples.
from sklearn import metrics
test_y = test_data_frame['classification'].map(classification_map)
accuracy_score = metrics.accuracy_score(test_y, predictions)
In our example, the accuracy_score returns the value of 0.856212763344 which can be interpreted as "~85% of predictions are correct".
Training Machine Learning Algorithms
Having the input data pre-processed, you're ready to provide it to the chosen algorithm in order to train the model.
In our presented example we decided to use the Multi-layer Perceptron (MLP) algorithm, which is an example of a supervised learning neural network classification algorithm. We won't be focusing on the details of the algorithm selection process in this article, however you should be aware that it depends on the type of problem you need to solve and the type and volume of data you possess.
A supervised learning algorithm is an approach that requires the training data to contain both the input object (a vector of features) and the expected output value for this object. Thus, we need to split our train_data_frame into:
- train_X – a DataFrame object containing input records with the classification column omitted
- train_y – a Series object containing only the classification column (mapped into boolean values)
classification_map = { '< =50K': True, '>50K': False }
train_X = train_data_frame[train_data_frame.columns.drop(‘classification')] train_y = train_data_frame[‘classification'].map(classification_map)
The classifier object (sklearn.neural_network.MLPClassifier) must be initialized with a number of parameters, such as the number of hidden layers of the neural network or their sizes (i.e. the number of neurons in each layer). For the sake of conciseness, we don't show how to determine the best values for those parameters. Take our word for it that the best accuracy for this problem can be achieved by a neural network consisting of 1 hidden layer containing 20 neurons.
from sklearn.neural_network import MLPClassifier
NUMBER_OF_LAYERS = 1 NEURONS_PER_LAYER = 20
classifier = MLPClassifier( hidden_layer_sizes=(NEURONS_PER_LAYER, ) * NUMBER_OF_LAYERS, alpha=0.01, random_state=1 )
Finally, we apply the training data to the classifier algorithm. Before we do that, we use our previously constructed mapper to transform the input the data into the numeric form to be understood by the classifier object.
from sklearn.pipeline import Pipeline
pipeline = Pipeline([ (‘mapper', mapper), (‘classifier', classifier) ])
model = pipeline.fit(X=train_X, y=train_y)
According to the scikit-learn documentation – all supervised estimators implement a fit(X, y) method to fit (train) the model and a predict(X) method that, given unlabeled observations X, returns the predicted labels y.
Machine Learning with Python is a favored choice for enthusiasts and professionals due to its extensive libraries and community support.
Model Selection and Hyperparameter Tuning
Selecting the right Machine Learning model and tuning its hyperparameters is crucial to achieving good performance. Here are some common techniques used for model selection and hyperparameter tuning:
- Cross-Validation: Cross-validation involves splitting the data into training and testing sets multiple times and evaluating the model’s performance on each split. This helps in assessing the model’s generalizability and robustness.
- Grid Search: Grid search involves trying out different combinations of hyperparameters and evaluating the model’s performance on a validation set. It systematically searches through a predefined set of hyperparameters to find the best combination.
- Random Search: Random search involves randomly sampling hyperparameters and evaluating the model’s performance on a validation set. It is often more efficient than grid search, especially when dealing with a large number of hyperparameters.
- Bayesian Optimization: Bayesian optimization uses Bayesian methods to search for the optimal hyperparameters. It builds a probabilistic model of the objective function and uses it to select the most promising hyperparameters to evaluate.
These techniques help in fine-tuning ML models to achieve the best possible performance.
Predicting Using the Model
The classification model produced as a result of the training process can be now used to predict the classification on the test set DataFrame or possibly totally new data out in the wild.
test_X = test_data_frame[test_data_frame.columns.drop('classification')] predictions = model.predict(X=test_X)
Evaluating the Model
The last step you should take is model evaluation. This will tell you how accurate the predictions made by the trained model are.
As you might notice, the evaluation process is executed on the previously extracted test set (test_X, test_y) which was not seen by the model earlier, during the training process.
You should never evaluate the model on the train set, because the results obtained would not translate into real world applications (in that way you wouldn't be able to verify if your model is able to make generalizations).
There are a couple of metrics that let you evaluate the accuracy of your model. The most basic one is sklearn.metrics.accuracy_score which represents a ratio of all correctly predicted values to all processed samples.
from sklearn import metrics
test_y = test_data_frame['classification'].map(classification_map)
accuracy_score = metrics.accuracy_score(test_y, predictions)
In our example, the accuracy_score returns the value of 0.856212763344 which can be interpreted as "~85% of predictions are correct".
Model Evaluation Metrics
Evaluating the performance of a Machine Learning model is crucial to ensure that it is working as expected. Here are some common metrics used to evaluate Machine Learning models:
- Accuracy: Accuracy measures the proportion of correctly classified instances out of all instances in the test dataset. It is a straightforward metric but may not be suitable for imbalanced datasets.
- Precision: Precision measures the proportion of true positives out of all positive predictions made by the model. It is particularly useful when the cost of false positives is high.
- Recall: Recall measures the proportion of true positives out of all actual positive instances in the test dataset. It is important when the cost of false negatives is high.
- F1 Score: The F1 score is the harmonic mean of precision and recall. It provides a balanced measure of a model’s performance, especially when dealing with imbalanced datasets.
- Mean Squared Error (MSE): MSE measures the average squared difference between predicted and actual values. It is commonly used for regression tasks to evaluate the accuracy of continuous predictions.
These metrics provide valuable insights into the performance of ML models, helping to identify areas for improvement.
Advanced Techniques for Machine Learning Models
As you delve deeper into machine learning, you’ll encounter advanced techniques that can significantly enhance model performance and handle more complex data. Here are some of the key advanced techniques:
Deep Learning: Deep learning, a subset of machine learning, involves neural networks with multiple layers. Techniques such as convolutional neural networks (CNNs) and recurrent neural networks (RNNs) are used for tasks like image and speech recognition, and natural language processing. These models can learn intricate patterns in data, making them suitable for complex tasks.
Ensemble Methods: Ensemble methods, such as bagging and boosting, combine multiple models to improve overall performance. Bagging involves training multiple models in parallel and averaging their predictions, while boosting trains models sequentially, with each model focusing on the errors of the previous ones. These methods can enhance model accuracy and robustness.
Transfer Learning: Transfer learning leverages pre-trained models as a starting point for new models. This approach is particularly useful when you have limited data, as it allows you to build on existing knowledge. For example, a pre-trained image recognition model can be fine-tuned for a specific task, reducing development time and improving performance.
Hyperparameter Tuning: Hyperparameter tuning involves adjusting model parameters to optimize performance. Techniques such as grid search and random search systematically explore different combinations of hyperparameters to find the best configuration. This process is crucial for maximizing the accuracy and efficiency of machine learning models.
By incorporating these advanced techniques, you can tackle more complex data, improve model performance, and reduce development time, making your machine learning projects more effective and efficient.
Real-World Applications of Machine Learning
Machine learning has a wide range of real-world applications that demonstrate its power and versatility. Here are some notable examples:
Image Recognition: Machine learning is extensively used in image recognition tasks, such as object detection and image classification. Applications include self-driving cars, where models identify and respond to objects on the road, and medical diagnosis, where models analyze medical images to detect diseases.
Natural Language Processing (NLP): NLP involves using machine learning to understand and generate human language. Applications include text classification, sentiment analysis, and language translation. For instance, chatbots and virtual assistants use NLP to interact with users, while language translation software helps break down language barriers.
Predictive Maintenance: In industries like manufacturing and transportation, machine learning models predict equipment failures and schedule maintenance before issues arise. This predictive maintenance approach reduces downtime and maintenance costs, improving operational efficiency.
Recommendation Systems: Machine learning powers recommendation systems that suggest products, services, or content based on user preferences. E-commerce platforms use these systems to recommend products, while media services suggest movies or music based on user behavior.
These applications highlight the transformative impact of machine learning in various fields, solving real-world problems and enhancing user experiences.
Troubleshooting and Debugging
Troubleshooting and debugging are essential skills for machine learning developers, as they help identify and resolve issues with models, data, and code. Here are some common techniques:
Data Visualization: Visualizing data can reveal issues with data quality, distribution, and relationships. Tools like matplotlib and seaborn help create visual representations of data, making it easier to spot anomalies and patterns.
Model Evaluation: Evaluating model performance is crucial for identifying issues like overfitting, underfitting, and bias. Metrics such as accuracy, precision, recall, and F1 score provide insights into how well a model performs and where improvements are needed.
Code Review: Reviewing code helps identify syntax errors, logical errors, and performance issues. Peer reviews and automated code analysis tools can ensure code quality and maintainability.
Debugging Tools: Debugging tools, such as print statements and interactive debuggers, help track down issues in code execution and model behavior. These tools allow developers to step through code, inspect variables, and understand the flow of execution.
By employing these troubleshooting and debugging techniques, developers can quickly identify and fix issues, improving model performance and reducing development time. These skills are crucial for ensuring the success of machine learning projects.
Summary: Why Python is the Top Choice for ML
We showed you how to run your first Machine Learning algorithm on an example dataset. By evaluating the created model we proved that Machine Learning works (85% accuracy is not a bad result).
What you should have noticed throughout the article is that we didn't write that much code. Certainly we didn't have to write the ML algorithm itself.
For each task along the way we had a ready-to-use, battle-tested Python library to do the heavy lifting for us:
- pandas for loading and playing around with data,
- matplotlib for visualizing the data,
- sklearn-pandas for transforming our inputs into a numerical matrix,
- sklearn for the actual ML and assessment.
What we had to write was just the glue-code that tied everything together.
And that's why Python is the number one language for doing Machine Learning – all the tools are there, the usage is simple, the documentation extensive and the community vibrant. You can have a Machine Learning solution running in no time!
Next Steps in Machine Learning
Once you have learned the basics of machine learning, there are several next steps you can take to further your knowledge and skills:
- Learning Advanced ML Techniques: Delve deeper into advanced topics such as deep learning, natural language processing, and computer vision. These areas offer exciting opportunities to solve complex problems using machine learning.
- Working on Real-World Projects: Apply ML techniques to real-world problems and datasets. This hands-on experience is invaluable for understanding the practical challenges and nuances of implementing Machine Learning solutions.
- Staying Up-to-Date with Industry Trends: Follow industry leaders and researchers to stay informed about the latest developments in ML. Reading research papers, attending conferences, and participating in online forums can help you stay current.
- Pursuing a Career in Machine Learning: Explore job opportunities in artificial intelligence and ML and develop the skills and knowledge required to succeed in the field. Building a strong portfolio of projects and gaining experience through internships or collaborations can enhance your career prospects.
By taking these steps, you can continue to grow and excel in the dynamic field of Machine Learning.
Appendix: The Complete ML script
Below you can find the complete ML script used for this article.
import csv import functools import os.path
import numpy from sqlalchemy import create_engine import pandas from sklearn_pandas import DataFrameMapper import sklearn.preprocessing from sklearn.pipeline import Pipeline from sklearn.neural_network import MLPClassifier from sklearn import metrics
DIR = '/home/user/workspace/machine-learning'
TRAIN_DATA_FILE_PATH = os.path.join(DIR, 'data', 'adult.data') TEST_DATA_FILE_PATH = os.path.join(DIR, 'data', 'adult.test')
TRAIN_DB_FILE_PATH = os.path.join(DIR, 'db', 'data.sqlite') TEST_DB_FILE_PATH = os.path.join(DIR, 'db', 'test.sqlite')
train_engine = create_engine(f'sqlite:///{TRAIN_DB_FILE_PATH}') test_engine = create_engine(f'sqlite:///{TEST_DB_FILE_PATH}')
INT = 'INTEGER' STR = 'VARCHAR'
FIELDS = ( ('age', INT), ('workclass', STR), ('fnlwgt', INT), ('education', STR), ('education_num', INT), ('marital_status', STR), ('occupation', STR), ('relationship', STR), ('race', STR), ('sex', STR), ('capital_gain', INT), ('capital_loss', INT), ('hours_per_week', INT), ('native_country', STR), ('classification', STR) )
def create_schema(connection): fields_sql = ', '.join( f'{field_name} {field_type}' for (field_name, field_type) in FIELDS ) connection.execute( f'CREATE TABLE adult (id INTEGER PRIMARY KEY, {fields_sql})' )
def read_data(data_file_path): with open(data_file_path, newline='') as csvfile: reader = csv.reader(csvfile, delimiter=',', skipinitialspace=True) for row in reader: if len(row) != 15: continue # Skip empty rows, skip test file header classification = row[-1] if classification.endswith('.'): # Test file has dots ('.') at the end of lines, strip them out. row[-1] = classification[:-1] yield row
def insert_row(row, connection): fields = ', '.join(field_name for (field_name, _) in FIELDS) placeholders = ', '.join(['?'] * len(FIELDS)) connection.execute( f'INSERT INTO adult ({fields}) VALUES ({placeholders})', row )
def import_data(data, connection): create_schema(connection) with connection.begin(): for row in data: insert_row(row, connection)
def gather_data(): return read_data(TRAIN_DATA_FILE_PATH), read_data(TEST_DATA_FILE_PATH)
def store_data(train_data, test_data): with train_engine.connect() as conn: import_data(train_data, conn) with test_engine.connect() as conn: import_data(test_data, conn)
def load_data(train_engine, test_engine): with train_engine.connect() as conn: with conn.begin(): train_data_frame = pandas.read_sql_table( 'adult', conn, index_col='id' )
with test_engine.connect() as conn: with conn.begin(): test_data_frame = pandas.read_sql_table( 'adult', conn, index_col='id' )
return train_data_frame, test_data_frame
def get_mapper(): def numpy_map(callback): @functools.wraps(callback) def numpy_map_wrapper(X): return numpy.array([callback(x) for x in X]) return numpy_map_wrapper
@numpy_map def native_country_generalize(x): return 'US' if x == 'United-States' else 'Other'
@numpy_map def workclass_generalize(x): if x in ['Self-emp-not-inc', 'Self-emp-inc']: return 'Self-emp' elif x in ['Local-gov', 'State-gov', 'Federal-gov']: return 'Gov' elif x in ['Without-pay', 'Never-worked', '?']: return 'None' else: return x
@numpy_map def education_generalize(x): if x in ['Assoc-voc', 'Assoc-acdm']: return 'Assoc' elif x in [ '11th', '10th', '7th-8th', '9th', '12th', '5th-6th', '1st-4th', 'Preschool' ]: return 'Low' else: return x
return DataFrameMapper([ (['age'], sklearn.preprocessing.StandardScaler()), ('workclass', [ sklearn.preprocessing.FunctionTransformer( workclass_generalize, validate=False ), sklearn.preprocessing.LabelBinarizer() ]), # ('fnlwgt', None), ('education', [ sklearn.preprocessing.FunctionTransformer( education_generalize, validate=False ), sklearn.preprocessing.LabelBinarizer() ]), (['education_num'], sklearn.preprocessing.StandardScaler()), ('marital_status', sklearn.preprocessing.LabelBinarizer()), ('occupation', sklearn.preprocessing.LabelBinarizer()), ('relationship', sklearn.preprocessing.LabelBinarizer()), ('race', sklearn.preprocessing.LabelBinarizer()), ('sex', sklearn.preprocessing.LabelBinarizer()), (['capital_gain'], sklearn.preprocessing.StandardScaler()), (['capital_loss'], sklearn.preprocessing.StandardScaler()), (['hours_per_week'], sklearn.preprocessing.StandardScaler()), ('native_country', [ sklearn.preprocessing.FunctionTransformer( native_country_generalize, validate=False ), sklearn.preprocessing.LabelBinarizer() ]), ])
classification_map = { '<=50K': True, '>50K': False }
def train(train_data_frame, mapper): train_X = train_data_frame[train_data_frame.columns.drop('classification')] train_y = train_data_frame['classification'].map(classification_map)
NUMBER_OF_LAYERS = 1 NEURONS_PER_LAYER = 20
classifier = MLPClassifier( hidden_layer_sizes=(NEURONS_PER_LAYER, ) * NUMBER_OF_LAYERS, alpha=0.01, random_state=1 )
pipeline = Pipeline([ ('mapper', mapper), ('classifier', classifier) ])
model = pipeline.fit(X=train_X, y=train_y) return model
def predict(model, test_data_frame): test_X = test_data_frame[test_data_frame.columns.drop('classification')]
predictions = model.predict(X=test_X) return predictions
def assess(test_data_frame, predictions): test_y = test_data_frame['classification'].map(classification_map)
accuracy_score = metrics.accuracy_score(test_y, predictions) return accuracy_score
def main(): train_data, test_data = gather_data() store_data(train_data, test_data) train_data_frame, test_data_frame = load_data(train_engine, test_engine) mapper = get_mapper() model = train(train_data_frame, mapper) predictions = predict(model, test_data_frame) score = assess(test_data_frame, predictions) print('Accuracy score', score)
if __name__ == '__main__': main()
cycler==0.10.0 matplotlib==2.1.1 numpy==1.13.3 pandas==0.21.1 pyparsing==2.2.0 python-dateutil==2.6.1 pytz==2017.3 scikit-learn==0.19.1 scipy==1.0.0 six==1.11.0 sklearn==0.0 sklearn-pandas==1.6.0 SQLAlchemy==1.1.15