What is Generative AI?
Generative artificial intelligence (AI) is an innovative field focused on creating new content, such as text, images, and music, by learning from large datasets. Generative AI uses complex generative AI algorithms to discern patterns and autonomously generate new content. Generative AI tools are cutting-edge innovations that are making significant impacts across a variety of fields, redefining what artificial intelligence can achieve.

Deep Learning & Neural Networks
Deep learning represents an advanced subset of machine learning that harnesses the power of neural networks to analyze and process vast quantities of data. These neural networks are intricately designed to mimic the architecture of the human brain, featuring numerous interconnected layers that act similarly to neurons.
Each layer processes input data and passes the transformed data to the subsequent layer, with the complexity and abstraction of features increasing at each stage. This hierarchical structure enables deep learning generative AI models to excel in tasks that require sophisticated pattern recognition capabilities, such as image recognition, natural language processing, and language translation.
The depth and breadth of these networks empower them to discern nuanced patterns and relationships within the data, achieving remarkable levels of accuracy and performance that are continually pushing the boundaries of generative AI applications.
Machine Learning & AI Algorithms
These algorithms, through sophisticated data analysis, enable generative AI systems to learn patterns, relationships, and structures from vast amounts of past data, allowing them to make informed predictions and generate new, original content. They also utilize generative AI models. Generative AI platforms are among the best tools available for 2024, assisting in brainstorming creative ideas, generating images, and producing marketing copy efficiently.
At the core of this process are advanced techniques like neural networks, which mimic the human brain’s structure and function, and deep learning, which involves training models with multiple layers to progressively extract higher-level features from raw input. These methods are fundamental in the development of generative models, such as Generative Adversarial Networks (GANs) and Variational Autoencoders (VAEs), that are capable of creating remarkably realistic images and even music.
For instance, in natural language processing (NLP), machine learning algorithms underpin models like GPT-3, which can generate human-like text by predicting the likelihood of a sequence of words based on the context provided. This allows for applications such as automatic content generation, translation services, and sophisticated chatbots that can engage in meaningful conversations with users.
Key Features of Generative AI

One of the key features of generative AI is its ability to use generative models for various applications. These models are built using different types of machine learning techniques and neural networks. Let's explore each individual AI tool.
Large Language Models
Large language models are advanced generative AI models designed to understand and generate human language. They are used in natural language processing tasks like text generation, machine translation, and sentiment analysis. These models can generate human-like responses, making them invaluable for applications like chatbots and virtual assistants. These generative AI tools are constantly evolving and their popularity is rapidly growing.
Generative Adversarial Networks (GANs)
Generative adversarial networks (GANs) consist of two neural networks – a generator and a discriminator – that work together to create realistic content. The generator creates new data instances, while the discriminator evaluates their authenticity. This iterative process enables GANs to produce highly realistic images, making them one of the most popular generative AI tools.
They are working to eliminate one of the drawbacks of using generative ai – the fear of unauthentic artificial intelligence content.
Diffusion Models
Diffusion models are a sophisticated type of generative AI models that simulate the physical process of diffusion for data generation and analysis. They involve a forward process where data is progressively corrupted by noise and a reverse process that iteratively denoises the data to restore it to its original distribution. These models excel in generating high-quality images, simulating scientific phenomena, detecting anomalies, and synthesizing audio.
Despite their computational intensity and training complexity, diffusion models offer remarkable flexibility and output quality. These generative AI models are mostly used in fields ranging from physics and biology to finance and cybersecurity.
Popular Generative AI Tools
There are many generative AI tools designed for various applications, from creating text and images to generating music and videos. Let's look at some of these generative AI tools and their capabilities.
Text Generation and Natural Language Processing Tasks
Text generation tools use large language models to create coherent and contextually relevant text. They are used for various natural language processing tasks, including writing marketing copy, generating code snippets, and creative writing.
Just a few examples include OpenAI’s GPT-3 and Google's BERT, which are widely adopted for content generation. This generative AI tool is extremely beneficial for copywriters, marketers and the "creative" industries.
AI Tools for Image and Video Content Generation
Generative AI tools can automatically create images and videos, often used in marketing and entertainment. Tools like DALL-E and VQ-VAE are breakthroughs in generating images, capable of creating photorealistic images from textual descriptions. These tools are also used in video content creation, enabling brands to produce high-quality, engaging videos effortlessly.
Music and Creative Content Creation
Music generation tools such as Jukedeck and Amper Music are revolutionizing the music creation landscape by leveraging advanced AI algorithms and generative AI tools to produce customizable, royalty-free tracks. These platforms enable users to generate music that matches specific moods, themes, or project requirements, thus offering a high degree of personalization and convenience.
As a result, content creators, marketers, and video producers can effortlessly integrate these unique soundtracks into their projects, circumventing the often cost-prohibitive and time-consuming process of traditional music licensing. This technological advancement not only establishes generative AI's position in music production but also enhances the efficiency and creativity of multimedia content creation.
Applications of Generative AI
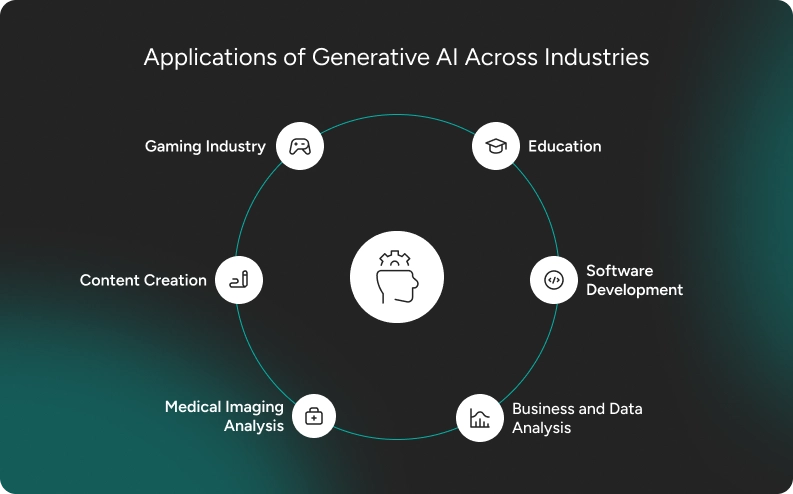
Generative AI is being applied in numerous fields, revolutionizing traditional processes and introducing new efficiencies. Using generative AI can enhance productivity and creativity across different fields such as marketing, sales, and product development. Let’s explore how each generative AI tool is used in different industries and for different causes.
Content Creation
Generative AI is widely used for content creation, including generating marketing copy, blog posts, and creative writing. AI-generated content can enhance productivity by automating repetitive tasks, allowing creators to focus on more strategic aspects. This is great for speeding up content generation, and image generation and making AI tools more accessible.
Software Development
Generative AI significantly enhances software development by automating tasks such as code generation and debugging. These AI models can produce code snippets tailored to specific functionalities and support multiple programming languages, streamlining cross-platform development.
Additionally, they possess the capability to write complete programs based on high-level requirements, drastically reducing the time and effort needed for coding. This accelerates the entire development lifecycle, from ideation to deployment, and allows developers to focus more on creative problem-solving and less on routine tasks.
Medical Imaging Analysis
In healthcare, generative AI plays a significant role in medical imaging analysis. AI models generate synthetic data that enhance the training of other models, bolstering their ability to accurately diagnose conditions. This is especially vital in radiology, where quick and precise image analysis is critical.
By simulating diverse medical scenarios, generative AI improves the diagnostic accuracy and efficiency of imaging tools, potentially leading to life-saving interventions. This generative AI tool is transforming a far from software industry - showing just how important it is to familiarize the world with generative AI.
Business and Data Analysis
Generative AI significantly transforms business processes by automating critical aspects of data analysis and decision-making, allowing organizations to operate with unprecedented efficiency and accuracy. By leveraging sophisticated generative AI tools, businesses can sift through complex and vast datasets, extracting valuable insights that inform and shape strategic decisions.
Such automation not only speeds up the analytical process but also enhances the precision and depth of the insights generated, enabling more informed and effective decision-making. In particular, sectors such as finance and marketing benefit immensely; AI-generated content and intelligent algorithms personalize customer interactions and experiences, tailoring communications and offerings to individual preferences and behaviors.
This personalization fosters deeper customer engagement and loyalty, driving better business outcomes. Consequently, generative AI doesn't just augment existing processes but also propels innovation, giving businesses a competitive edge in the marketplace.
Gaming Industry
Generative AI is transforming the gaming industry by automating the creation of game assets, environments, and even narratives. Procedural content generation can create expansive and diverse game worlds, making each gaming experience unique. AI can also drive non-playable characters (NPCs) with realistic behaviors and dialogues, enhancing player immersion.
Generative models are also being used to write game scripts and can even provide multiple endings based on the player's choices.
Education
Generative models are reshaping education by providing personalized learning experiences and generating educational content. AI-driven platforms can create quizzes, summaries, and even entire course modules tailored to individual learning styles, helping students grasp complex subjects more effectively.
Schools are also taking action and going with the flow, creating AI courses and informing their students on the importance of taking precautions and being mindful while using AI tools.
Benefits and Challenges
While generative AI offers numerous benefits, it also comes with its set of challenges.
Advantages
Generative AI has the potential to revolutionize efficiency and creativity across various sectors by enabling rapid content creation and massive scalability. AI-driven systems can quickly produce diverse types of content – including text, images, videos, music, and code – far faster than human capabilities permit.
Models like GPT-4 can generate articles, blog posts, or social media updates in seconds, drastically reducing the time from concept to final product. This automation also allows businesses to scale their content production without a corresponding increase in labor costs, thereby significantly streamlining operations.
By generating multiple pieces of content simultaneously, companies can greatly expand their outreach and engagement efforts efficiently, making AI a valuable asset for modern enterprises.
Challenges
Despite its advantages, generative AI faces ethical concerns and challenges related to the quality of its training data. Ensuring that AI models are trained on diverse, high-quality data is crucial for their effectiveness and fairness. Poor-quality or biased data can lead to inaccurate, harmful, or ethically problematic outputs.
Moreover, ongoing AI research is essential to address these ethical issues and enhance the capabilities of generative AI. Researchers and developers must continuously work to improve the transparency, accountability, and inclusivity of AI systems, ensuring that they benefit society as a whole while mitigating potential risks and biases. Generative AI tools being so accessible can also result in content generation that spreads fake news and misinformation, and as of recently, these tools can be utilized to create harmful content featuring real people.
Recent society's uproar was well justified, as celebrities are being tackled and generative models are used to create synthetic data consisting of their appearances, portraying them in fake and hurtful situations. It will not take long before regular people become the target if certain regulations and laws will not be applied.
Future of Generative AI
The future of generative AI holds great promise, featuring a variety of emerging trends and potential innovations on the horizon. As the technology continues to evolve, we can anticipate more sophisticated models capable of producing highly realistic images, text, and even complex simulations.
Innovations in areas like natural language processing, creative content generation, and automated decision-making are likely to revolutionize industries ranging from entertainment and media to healthcare and finance.
Emerging Trends
The development of foundation models, which serve as the basis for various AI technologies, represents an exciting and transformative trend in the field of artificial intelligence. These models, often characterized by their massive scale and versatility, are becoming increasingly sophisticated, enabling more advanced and diverse applications of generative AI.
Foundation models like GPT-3, BERT, and DALL-E are pre-trained on vast datasets and have the capability to understand and generate human-like text, create astonishingly realistic images, and perform a multitude of other tasks with high accuracy. Their adaptability means they can be fine-tuned for specific applications across different domains, from natural language processing and automated content creation to scientific research and personalized healthcare.
The increasing complexity and performance of these models are underpinned by advances in machine learning algorithms, greater computational resources, and an expanding volume of training data. Such developments are pushing the boundaries of what generative AI can achieve, making it a crucial tool for innovation in various fields.
For example, in drug discovery, foundation models can predict molecular interactions and generate potential new compounds, significantly accelerating the identification of viable medications. In the creative industries, they enable artists and designers to explore new forms of expression and generate unique content that merges human creativity with machine intelligence.
Moreover, with ongoing research focused on improving the interpretability and ethical considerations of these models, there is a growing emphasis on ensuring that the deployment of generative AI is responsible and beneficial to society. As foundation models continue to evolve, they are expected to integrate seamlessly with other advanced technologies like edge computing, blockchain, and the Internet of Things (IoT), further expanding their applications and impact.
This confluence of factors positions foundation models as a cornerstone in the future landscape of artificial intelligence, driving forward an era of unprecedented innovation and possibility.
Potential Innovations
Future innovations in generative AI are expected to bring significant improvements in human-like responses and more complex data-processing AI capabilities, broadening the scope of its applications across a diverse array of fields and industries.
As AI systems become better at understanding and mimicking human language, emotions, and behaviors, we can anticipate more natural and intuitive interactions between humans and machines. This will enhance the user experience in everything from customer service and virtual assistants to support and education, where AI can provide empathetic and contextually appropriate responses.
Furthermore, advancements in data processing will enable generative AI to handle increasingly complex and nuanced information, allowing it to perform tasks that require deep understanding and sophisticated analysis. For instance, in the field of medicine, AI could analyze vast amounts of patient data to provide more accurate diagnoses, and personalized treatment plans, and even predict outbreaks of diseases. In the financial sector, more advanced generative AI could offer enhanced risk assessment, fraud detection, and personalized financial advice by processing and interpreting complex market data and economic indicators.
The integration of these sophisticated capabilities will open up new possibilities for innovation and efficiency across many industries. For example, in creative fields such as film, music, and literature, AI could collaborate with human creators to produce original content, offering novel ideas, image generation and speeding up the creative process. In scientific research, AI could generate hypotheses, design experiments, and even analyze experimental data, significantly accelerating the pace of discovery.
As the technology continues to advance, ethical considerations and regulations will be crucial to ensure that these powerful tools are used responsibly and for the benefit of society. Efforts to improve transparency, fairness, and accountability in AI systems will help build trust and ensure that the deployment of generative AI is aligned with human values.
As a result, the future of generative AI promises to be one of not only technological sophistication but also ethical and socially aware innovation, transforming the way we live and work in ways that are beyond our current imagination.
Generative AI: What's Next?
Generative AI has transformative potential, revolutionizing industries and introducing new efficiencies. From content creation to medical imaging analysis, generative AI models enhance human intelligence with machine learning and AI algorithms, making significant impacts. As businesses evaluate their current AI strategies, further exploration and investment in AI research are essential.